借助 Visual Question Answering (VQA),您可以向模型提供图片,并提出有关图片内容的问题。在问题的响应中,您会获得一个或多个自然语言回答。
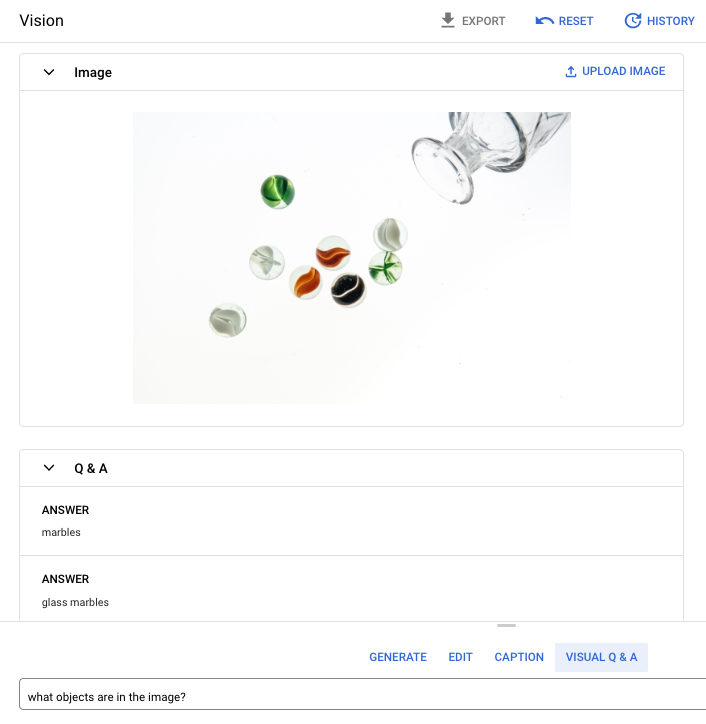
提示问题:图片中包含哪些对象?
回答1:弹珠
回答 2:玻璃弹珠
支持的语言
VQA 支持以下语言:
- 英语 (en)
性能和限制
使用此模型时,存在以下限制:
限制 | 值 |
---|---|
每项目每分钟的 API 请求(短)数上限 | 500 |
响应(短)中返回的词元数上限 | 64 个词元 |
请求(仅限短 VQA)中接受的词元数上限 | 80 个词元 |
使用此模型时,预计会有以下服务延迟时间。这些值仅作说明之用,并非服务承诺:
延迟时间 | 值 |
---|---|
API 请求(短) | 1.5 秒 |
位置
位置是您可以在请求中指定的区域,用于控制静态数据的存储位置。如需查看可用区域的列表,请参阅 Vertex AI 上的生成式 AI 位置。
Responsible AI 安全过滤
图片标注和 Visual Question Answering (VQA) 特征模型不支持用户可配置的安全过滤器。但是,整体 Imagen 安全过滤会对以下数据进行:
- 用户输入
- 模型输出
因此,如果 Imagen 应用这些安全过滤器,您的输出可能会与示例输出有所不同。请参考以下示例。
过滤后的输入
如果输入已过滤,则回答类似于以下内容:
{
"error": {
"code": 400,
"message": "Media reasoning failed with the following error: The response is blocked, as it may violate our policies. If you believe this is an error, please send feedback to your account team. Error Code: 63429089, 72817394",
"status": "INVALID_ARGUMENT",
"details": [
{
"@type": "type.googleapis.com/google.rpc.DebugInfo",
"detail": "[ORIGINAL ERROR] generic::invalid_argument: Media reasoning failed with the following error: The response is blocked, as it may violate our policies. If you believe this is an error, please send feedback to your account team. Error Code: 63429089, 72817394 [google.rpc.error_details_ext] { message: \"Media reasoning failed with the following error: The response is blocked, as it may violate our policies. If you believe this is an error, please send feedback to your account team. Error Code: 63429089, 72817394\" }"
}
]
}
}
过滤后的输出
如果返回的回答数量小于您指定的样本数量,这意味着缺失的回答已被 Responsible AI 过滤。例如,以下是对具有 "sampleCount": 2
的请求的回答,但其中一个回答已被过滤掉:
{
"predictions": [
"cappuccino"
]
}
如果所有输出都经过过滤,则回答是一个类似于以下内容的空对象:
{}
对图片使用 VQA(短响应)
以下示例展示了如何提出有关图片的问题并获得回答。
REST
如需详细了解 imagetext
模型请求,请参阅 imagetext
模型 API 参考文档。
在使用任何请求数据之前,请先进行以下替换:
- PROJECT_ID:您的 Google Cloud 项目 ID。
- LOCATION:您的项目的区域。 例如
us-central1
、europe-west2
或asia-northeast3
。如需查看可用区域的列表,请参阅 Vertex AI 上的生成式 AI 位置。 - VQA_PROMPT:有关图片的问题,您希望获得该问题的回答。
- 这只鞋是什么颜色的?
- 衬衫上用的是哪种类型的袖套?
- B64_IMAGE:要获取其说明的图片。图片必须指定为 base64 编码的字节字符串。大小上限:10 MB。
- RESPONSE_COUNT:您要生成的回答数量。接受的整数值:1-3。
HTTP 方法和网址:
POST https://LOCATION-aiplatform.googleapis.com/v1/projects/PROJECT_ID/locations/LOCATION/publishers/google/models/imagetext:predict
请求 JSON 正文:
{ "instances": [ { "prompt": "VQA_PROMPT", "image": { "bytesBase64Encoded": "B64_IMAGE" } } ], "parameters": { "sampleCount": RESPONSE_COUNT } }
如需发送请求,请选择以下方式之一:
curl
将请求正文保存在名为 request.json
的文件中,然后执行以下命令:
curl -X POST \
-H "Authorization: Bearer $(gcloud auth print-access-token)" \
-H "Content-Type: application/json; charset=utf-8" \
-d @request.json \
"https://LOCATION-aiplatform.googleapis.com/v1/projects/PROJECT_ID/locations/LOCATION/publishers/google/models/imagetext:predict"
PowerShell
将请求正文保存在名为 request.json
的文件中,然后执行以下命令:
$cred = gcloud auth print-access-token
$headers = @{ "Authorization" = "Bearer $cred" }
Invoke-WebRequest `
-Method POST `
-Headers $headers `
-ContentType: "application/json; charset=utf-8" `
-InFile request.json `
-Uri "https://LOCATION-aiplatform.googleapis.com/v1/projects/PROJECT_ID/locations/LOCATION/publishers/google/models/imagetext:predict" | Select-Object -Expand Content
"sampleCount": 2
和 "prompt": "What is this?"
的请求。该响应会返回两个预测字符串答案。
{ "predictions": [ "cappuccino", "coffee" ] }
Python
在尝试此示例之前,请按照《Vertex AI 快速入门:使用客户端库》中的 Python 设置说明执行操作。 如需了解详情,请参阅 Vertex AI Python API 参考文档。
如需向 Vertex AI 进行身份验证,请设置应用默认凭据。 如需了解详情,请参阅为本地开发环境设置身份验证。
在此示例中,您将使用 load_from_file
方法引用本地文件作为基础 Image
,以获取相关信息。指定基础图片后,您可以对 ImageTextModel
使用 ask_question
方法并输出答案。
Node.js
在尝试此示例之前,请按照《Vertex AI 快速入门:使用客户端库》中的 Node.js 设置说明执行操作。 如需了解详情,请参阅 Vertex AI Node.js API 参考文档。
如需向 Vertex AI 进行身份验证,请设置应用默认凭据。 如需了解详情,请参阅为本地开发环境设置身份验证。
在此示例中,您将对PredictionServiceClient
调用 predict
方法。
该服务会返回所提供问题的答案。
为 VQA 使用参数
获得 VQA 回复时,您可以根据自己的应用场景设置多个参数。
结果数量
使用结果数参数来限制为您发送的每个请求返回的回复数量。如需了解详情,请参阅 imagetext
(VQA) 模型 API 参考文档。
种子编号
您为请求添加的数字,以使生成的图片回复具有确定性。通过在请求中添加种子编号,可确保您每次都获得相同的预测结果(回复)。但是,回答不一定以相同顺序返回。如需了解详情,请参阅 imagetext
(VQA) 模型 API 参考文档。
后续步骤
阅读有关 Imagen 和其他 Vertex AI 上的生成式 AI 产品的文章:
- Imagen 3 on Vertex AI 开发者入门指南
- 与创作者一起为创作者打造的全新生成式媒体模型和工具
- Gemini 中的新功能:自定义 Gem 以及通过 Imagen 3 改进的图片生成
- Google DeepMind:Imagen 3 - 我们质量最高的文本转图片模型