このページでは、Dataflow パイプラインの作成または実行中に問題が発生した場合に役立つ、トラブルシューティングのヒントとデバッグ方法を説明します。この情報は、パイプラインの障害の検出、パイプラインの実行に失敗した理由の判別、問題を解決するための一連のアクションの提案を支援します。
次の図は、このページで説明する Dataflow トラブルシューティングのワークフローを示しています。
Dataflow にはジョブについてのリアルタイム フィードバック機能とともに、エラー メッセージ、ログ、およびジョブの進捗が円滑でないなどの状態をチェックするために使用できる一連の基本ステップが用意されています。
Dataflow ジョブの実行時に発生する可能性のある一般的なエラーに関するガイダンスについては、Dataflow エラーのトラブルシューティングをご覧ください。パイプライン パフォーマンスのモニタリングとトラブルシューティングについては、パイプライン パフォーマンスのモニタリングをご覧ください。
パイプラインのベスト プラクティス
Java、Python、Go パイプラインのベスト プラクティスは次のとおりです。
Java
Python
一時的なロケーションとステージング ロケーションの両方に接頭辞 <job_name>.<time>
が付きます。
必ず、ステージング ロケーションと一時的なロケーションの両方を異なるロケーションに設定してください。
必要に応じて、ジョブが完了または停止した後にステージング場所にあるオブジェクトを削除します。また、ステージングされたオブジェクトが Python パイプラインで再利用されることはありません。
ジョブが終了し、一時オブジェクトがクリーンアップされない場合は、一時的なロケーションとして使用されている Cloud Storage バケットからこれらのファイルを手動で削除します。
バッチジョブの場合、一時的なロケーションとステージング ロケーションの両方に有効期間(TTL)を設定することをおすすめします。
Go
一時的なロケーションとステージング ロケーションの両方に接頭辞
<job_name>.<time>
が付きます。必ず、ステージング ロケーションと一時的なロケーションの両方を異なるロケーションに設定してください。
必要に応じて、ジョブが完了または停止した後にステージング場所にあるオブジェクトを削除します。また、ステージングされたオブジェクトが Go パイプラインで再利用されることはありません。
ジョブが終了し、一時オブジェクトがクリーンアップされない場合は、一時的なロケーションとして使用されている Cloud Storage バケットからこれらのファイルを手動で削除します。
バッチジョブの場合、一時的なロケーションとステージング ロケーションの両方に有効期間(TTL)を設定することをおすすめします。
パイプラインのステータスをチェックする
Dataflow モニタリング インターフェースを使用して、パイプラインの実行のエラーを検出できます。
- Google Cloud コンソールに移動します。
- プロジェクト リストから Google Cloud プロジェクトを選択します。
- ナビゲーション メニューの [ビッグデータ] で、[Dataflow] をクリックします。実行中のジョブのリストが右側のペインに表示されます。
- 表示するパイプライン ジョブを選択します。ジョブのステータス概要が、[ステータス] フィールドに表示されます(実行中、完了、失敗)。
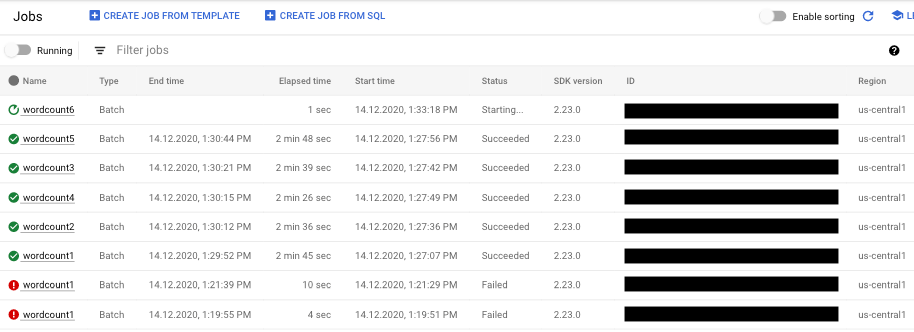
パイプラインの障害に関する情報を見つける
パイプライン ジョブの 1 つが失敗した場合は、ジョブを選択してエラーと実行結果の詳細情報を表示できます。ジョブを選択すると、パイプラインの主なグラフ、実行グラフ、[ジョブ情報] パネルと [ログ] パネルに、[ジョブのログ]、[ワーカーログ]、[診断]、[推奨事項] のタブが表示されます。
ジョブのエラー メッセージを確認する
パイプライン コードと Dataflow サービスによって生成されたジョブのログを表示するには、[ログ] パネルで [segment表示] をクリックします。
[情報] arrow_drop_down と filter_list [フィルタ] をクリックすると、[ジョブのログ] に表示されるメッセージをフィルタリングできます。エラー メッセージだけを表示するには、[情報] arrow_drop_down をクリックして、[エラー] を選択します。
エラー メッセージを展開するには、展開可能なセクション arrow_right をクリックします。
または、[診断] タブをクリックします。このタブには、選択したタイムラインでエラーが発生した場所、ログに記録されたすべてのエラーの数、パイプラインの推奨事項が表示されます。
ジョブのステップログを表示する
パイプライン グラフのステップを選択すると、ログパネルは、Dataflow サービスによって生成されたジョブログの表示から、パイプライン ステップを実行している Compute Engine インスタンスからのログの表示に切り替わります。
Cloud Logging は、プロジェクトの Compute Engine インスタンスから収集されたすべてのログを 1 か所にまとめます。Dataflow のさまざまなログ機能の使用について詳しくは、パイプライン メッセージをログに記録するをご覧ください。
自動化されたパイプラインの拒否を処理する
場合によっては、パイプラインで既知の SDK の問題がトリガーされる可能性があることを Dataflow サービスが認識することがあります。問題が発生する可能性が高いパイプラインが送信されないように、Dataflow はパイプラインを自動的に拒否して次のメッセージを表示します。
The workflow was automatically rejected by the service because it might trigger an identified bug in the SDK (details below). If you think this identification is in error, and would like to override this automated rejection, please re-submit this workflow with the following override flag: [OVERRIDE FLAG]. Bug details: [BUG DETAILS]. Contact Google Cloud Support for further help. Please use this identifier in your communication: [BUG ID].
リンクされているバグの詳細にある注意点を確認した後、なおパイプラインの実行を試みる場合は、自動拒否をオーバーライドできます。--experiments=<override-flag>
フラグを追加してパイプラインを再送信します。
パイプラインの失敗の原因を判別する
通常、Apache Beam パイプラインの実行の失敗は、次のいずれかが原因です。
- グラフまたはパイプラインの作成エラー。これらのエラーは、Dataflow が Apache Beam パイプラインの内容に従ってパイプラインを構成するステップのグラフを構築する際に問題が発生した場合に発生します。
- ジョブ検証のエラー。Dataflow サービスでは、開始したパイプライン ジョブが検証されます。検証プロセスのエラーにより、ジョブが正常に作成または実行されないことがあります。検証エラーには、Google Cloud プロジェクトの Cloud Storage バケットに関する問題や、プロジェクトの権限に関する問題が含まれることがあります。
- ワーカーコードの例外。これらのエラーは、
ParDo
変換のDoFn
インスタンスなど、ユーザーが提供し、Dataflow が並列ワーカーに配布するコードにエラーまたはバグがある場合に発生します。 - 他の Google Cloud サービスの一時的な障害が原因のエラー。Compute Engine や Cloud Storage など、Dataflow が依存する Google Cloud サービスの一時的な停止などの問題が原因でパイプラインが失敗することがあります。
グラフまたはパイプラインの作成エラーを検出する
グラフの作成エラーは、Dataflow が Dataflow プログラムのコードからパイプラインの実行グラフを作成している場合に発生することがあります。グラフの作成中に、Dataflow は無効なオペレーションをチェックします。
Dataflow がグラフの作成でエラーを検出した場合は、Dataflow サービスにジョブが作成されないことに注意してください。そのため、Dataflow モニタリング インターフェースにはフィードバックが表示されません。代わりに、Apache Beam パイプラインを実行したコンソールまたはターミナル ウィンドウに次のようなエラー メッセージが表示されます。
Java
たとえば、グローバルにウィンドウ処理され、トリガーされない制限なしの PCollection
で GroupByKey
のような集計を実行しようとすると、次のようなエラー メッセージが表示されます。
... ... Exception in thread "main" java.lang.IllegalStateException: ... GroupByKey cannot be applied to non-bounded PCollection in the GlobalWindow without a trigger. ... Use a Window.into or Window.triggering transform prior to GroupByKey ...
Python
たとえば、パイプラインがタイプヒントを使用し、変換の 1 つの引数タイプが期待されたものでない場合は、次のようなエラー メッセージが表示されます。
... in <module> run() ... in run | beam.Map('count', lambda (word, ones): (word, sum(ones)))) ... in __or__ return self.pipeline.apply(ptransform, self) ... in apply transform.type_check_inputs(pvalueish) ... in type_check_inputs self.type_check_inputs_or_outputs(pvalueish, 'input') ... in type_check_inputs_or_outputs pvalue_.element_type)) google.cloud.dataflow.typehints.decorators.TypeCheckError: Input type hint violation at group: expected Tuple[TypeVariable[K], TypeVariable[V]], got <type 'str'>
Go
たとえば、入力を受け取らない「DoFn」をパイプラインが使用すると、次のようなエラー メッセージが表示されます。
... panic: Method ProcessElement in DoFn main.extractFn is missing all inputs. A main input is required. ... Full error: ... inserting ParDo in scope root/CountWords ... graph.AsDoFn: for Fn named main.extractFn ... ProcessElement method has no main inputs ... goroutine 1 [running]: ... github.com/apache/beam/sdks/v2/go/pkg/beam.MustN(...) ... (more stacktrace)
このようなエラーが発生した場合は、パイプライン コードをチェックして、パイプラインのオペレーションが有効であることを確認してください。
Dataflow ジョブ検証でエラーを検出する
Dataflow サービスがパイプラインのグラフを受信すると、サービスはジョブの検証を試みます。この検証には以下の作業が含まれます。
- サービスがファイルのステージングおよび一時出力についてジョブに関連する Cloud Storage バケットにアクセスできることを確認します。
- Google Cloud プロジェクトで必要な権限を確認します。
- サービスがファイルなどの入出力ソースにアクセスできることを確認します。
ジョブが検証プロセスに失敗すると、Dataflow モニタリング インターフェースにエラー メッセージが表示されます。ブロック実行を使用している場合には、コンソールまたはターミナル ウィンドウにもエラー メッセージが表示されます。次のようなエラー メッセージが表示されます。
Java
INFO: To access the Dataflow monitoring console, please navigate to https://console.developers.google.com/project/google.com%3Aclouddfe/dataflow/job/2016-03-08_18_59_25-16868399470801620798 Submitted job: 2016-03-08_18_59_25-16868399470801620798 ... ... Starting 3 workers... ... Executing operation BigQuery-Read+AnonymousParDo+BigQuery-Write ... Executing BigQuery import job "dataflow_job_16868399470801619475". ... Stopping worker pool... ... Workflow failed. Causes: ...BigQuery-Read+AnonymousParDo+BigQuery-Write failed. Causes: ... BigQuery getting table "non_existent_table" from dataset "cws_demo" in project "my_project" failed. Message: Not found: Table x:cws_demo.non_existent_table HTTP Code: 404 ... Worker pool stopped. ... com.google.cloud.dataflow.sdk.runners.BlockingDataflowPipelineRunner run INFO: Job finished with status FAILED Exception in thread "main" com.google.cloud.dataflow.sdk.runners.DataflowJobExecutionException: Job 2016-03-08_18_59_25-16868399470801620798 failed with status FAILED at com.google.cloud.dataflow.sdk.runners.DataflowRunner.run(DataflowRunner.java:155) at com.google.cloud.dataflow.sdk.runners.DataflowRunner.run(DataflowRunner.java:56) at com.google.cloud.dataflow.sdk.Pipeline.run(Pipeline.java:180) at com.google.cloud.dataflow.integration.BigQueryCopyTableExample.main(BigQueryCopyTableExample.java:74)
Python
INFO:root:Created job with id: [2016-03-08_14_12_01-2117248033993412477] ... Checking required Cloud APIs are enabled. ... Job 2016-03-08_14_12_01-2117248033993412477 is in state JOB_STATE_RUNNING. ... Combiner lifting skipped for step group: GroupByKey not followed by a combiner. ... Expanding GroupByKey operations into optimizable parts. ... Lifting ValueCombiningMappingFns into MergeBucketsMappingFns ... Annotating graph with Autotuner information. ... Fusing adjacent ParDo, Read, Write, and Flatten operations ... Fusing consumer split into read ... ... Starting 1 workers... ... ... Executing operation read+split+pair_with_one+group/Reify+group/Write ... Executing failure step failure14 ... Workflow failed. Causes: ... read+split+pair_with_one+group/Reify+group/Write failed. Causes: ... Unable to view metadata for files: gs://dataflow-samples/shakespeare/missing.txt. ... Cleaning up. ... Tearing down pending resources... INFO:root:Job 2016-03-08_14_12_01-2117248033993412477 is in state JOB_STATE_FAILED.
Go
現在 Go では、このセクションで説明するジョブの検証はサポートされていません。こうした問題によるエラーは、ワーカー例外として現われます。
ワーカーコードで例外を検出する
ジョブの実行中に、ワーカーコードでエラーまたは例外が発生することがあります。これらのエラーは一般に、処理されない例外がパイプライン コードの DoFn
によって生成された結果、Dataflow ジョブのタスクが失敗したことを意味します。
ユーザーコード(DoFn
インスタンスなど)における例外が Dataflow モニタリング インターフェースで報告されます。ブロッキング実行でパイプラインを実行している場合、コンソールまたはターミナル ウィンドウに次のようなエラー メッセージが表示されます。
Java
INFO: To access the Dataflow monitoring console, please navigate to https://console.developers.google.com/project/example_project/dataflow/job/2017-05-23_14_02_46-1117850763061203461 Submitted job: 2017-05-23_14_02_46-1117850763061203461 ... ... To cancel the job using the 'gcloud' tool, run: gcloud beta dataflow jobs --project=example_project cancel 2017-05-23_14_02_46-1117850763061203461 ... Autoscaling is enabled for job 2017-05-23_14_02_46-1117850763061203461. ... The number of workers will be between 1 and 15. ... Autoscaling was automatically enabled for job 2017-05-23_14_02_46-1117850763061203461. ... ... Executing operation BigQueryIO.Write/BatchLoads/Create/Read(CreateSource)+BigQueryIO.Write/BatchLoads/GetTempFilePrefix+BigQueryIO.Write/BatchLoads/TempFilePrefixView/BatchViewOverrides.GroupByWindowHashAsKeyAndWindowAsSortKey/ParDo(UseWindowHashAsKeyAndWindowAsSortKey)+BigQueryIO.Write/BatchLoads/TempFilePrefixView/Combine.GloballyAsSingletonView/Combine.globally(Singleton)/WithKeys/AddKeys/Map/ParMultiDo(Anonymous)+BigQueryIO.Write/BatchLoads/TempFilePrefixView/Combine.GloballyAsSingletonView/Combine.globally(Singleton)/Combine.perKey(Singleton)/GroupByKey/Reify+BigQueryIO.Write/BatchLoads/TempFilePrefixView/Combine.GloballyAsSingletonView/Combine.globally(Singleton)/Combine.perKey(Singleton)/GroupByKey/Write+BigQueryIO.Write/BatchLoads/TempFilePrefixView/BatchViewOverrides.GroupByWindowHashAsKeyAndWindowAsSortKey/BatchViewOverrides.GroupByKeyAndSortValuesOnly/Write ... Workers have started successfully. ... ... org.apache.beam.runners.dataflow.util.MonitoringUtil$LoggingHandler process SEVERE: 2017-05-23T21:06:33.711Z: (c14bab21d699a182): java.lang.RuntimeException: org.apache.beam.sdk.util.UserCodeException: java.lang.ArithmeticException: / by zero at com.google.cloud.dataflow.worker.runners.worker.GroupAlsoByWindowsParDoFn$1.output(GroupAlsoByWindowsParDoFn.java:146) at com.google.cloud.dataflow.worker.runners.worker.GroupAlsoByWindowFnRunner$1.outputWindowedValue(GroupAlsoByWindowFnRunner.java:104) at com.google.cloud.dataflow.worker.util.BatchGroupAlsoByWindowAndCombineFn.closeWindow(BatchGroupAlsoByWindowAndCombineFn.java:191) ... ... Cleaning up. ... Stopping worker pool... ... Worker pool stopped.
Python
INFO:root:Job 2016-03-08_14_21_32-8974754969325215880 is in state JOB_STATE_RUNNING. ... INFO:root:... Expanding GroupByKey operations into optimizable parts. INFO:root:... Lifting ValueCombiningMappingFns into MergeBucketsMappingFns INFO:root:... Annotating graph with Autotuner information. INFO:root:... Fusing adjacent ParDo, Read, Write, and Flatten operations ... INFO:root:...: Starting 1 workers... INFO:root:...: Executing operation group/Create INFO:root:...: Value "group/Session" materialized. INFO:root:...: Executing operation read+split+pair_with_one+group/Reify+group/Write INFO:root:Job 2016-03-08_14_21_32-8974754969325215880 is in state JOB_STATE_RUNNING. INFO:root:...: ...: Workers have started successfully. INFO:root:Job 2016-03-08_14_21_32-8974754969325215880 is in state JOB_STATE_RUNNING. INFO:root:...: Traceback (most recent call last): File ".../dataflow_worker/batchworker.py", line 384, in do_work self.current_executor.execute(work_item.map_task) ... File ".../apache_beam/examples/wordcount.runfiles/py/apache_beam/examples/wordcount.py", line 73, in <lambda> ValueError: invalid literal for int() with base 10: 'www'
Go
... 2022-05-26T18:32:52.752315397Zprocess bundle failed for instruction ... process_bundle-4031463614776698457-2 using plan s02-6 : while executing ... Process for Plan[s02-6] failed: Oh no! This is an error message!
例外ハンドラを追加することでコード内のエラーから保護することを検討してください。たとえば、ParDo
で実行されたいくつかのカスタム入力検証が失敗する要素を削除する場合は、DoFn
内で例外を処理し、要素をドロップします。
いくつかの異なる方法で、失敗した要素を追跡することもできます。
- 失敗した要素をログに記録し、Cloud Logging を使用して出力をチェックできます。
- ログの表示の手順に沿って、Dataflow ワーカーログとワーカー起動ログで警告やエラーを確認できます。
- 後で検査できるように、
ParDo
に失敗した要素を追加出力へ書き込ませることができます。
実行中のパイプラインのプロパティを追跡するには、次の例のように Metrics
クラスを使用できます。
Java
final Counter counter = Metrics.counter("stats", "even-items"); PCollection<Integer> input = pipeline.apply(...); ... input.apply(ParDo.of(new DoFn<Integer, Integer>() { @ProcessElement public void processElement(ProcessContext c) { if (c.element() % 2 == 0) { counter.inc(); } });
Python
class FilterTextFn(beam.DoFn): """A DoFn that filters for a specific key based on a regex.""" def __init__(self, pattern): self.pattern = pattern # A custom metric can track values in your pipeline as it runs. Create # custom metrics to count unmatched words, and know the distribution of # word lengths in the input PCollection. self.word_len_dist = Metrics.distribution(self.__class__, 'word_len_dist') self.unmatched_words = Metrics.counter(self.__class__, 'unmatched_words') def process(self, element): word = element self.word_len_dist.update(len(word)) if re.match(self.pattern, word): yield element else: self.unmatched_words.inc() filtered_words = ( words | 'FilterText' >> beam.ParDo(FilterTextFn('s.*')))
Go
func addMetricDoFnToPipeline(s beam.Scope, input beam.PCollection) beam.PCollection { return beam.ParDo(s, &MyMetricsDoFn{}, input) } func executePipelineAndGetMetrics(ctx context.Context, p *beam.Pipeline) (metrics.QueryResults, error) { pr, err := beam.Run(ctx, runner, p) if err != nil { return metrics.QueryResults{}, err } // Request the metric called "counter1" in namespace called "namespace" ms := pr.Metrics().Query(func(r beam.MetricResult) bool { return r.Namespace() == "namespace" && r.Name() == "counter1" }) // Print the metric value - there should be only one line because there is // only one metric called "counter1" in the namespace called "namespace" for _, c := range ms.Counters() { fmt.Println(c.Namespace(), "-", c.Name(), ":", c.Committed) } return ms, nil } type MyMetricsDoFn struct { counter beam.Counter } func init() { beam.RegisterType(reflect.TypeOf((*MyMetricsDoFn)(nil))) } func (fn *MyMetricsDoFn) Setup() { // While metrics can be defined in package scope or dynamically // it's most efficient to include them in the DoFn. fn.counter = beam.NewCounter("namespace", "counter1") } func (fn *MyMetricsDoFn) ProcessElement(ctx context.Context, v beam.V, emit func(beam.V)) { // count the elements fn.counter.Inc(ctx, 1) emit(v) }
実行速度が遅いパイプラインまたは出力の欠落をトラブルシューティングする
遅いジョブや停止したジョブのトラブルシューティングをご覧ください。
一般的なエラーと一連のアクション
パイプラインのエラーの原因となった問題が判明している場合は、Dataflow エラーのトラブルシューティング ページでエラーのトラブルシューティング ガイダンスをご覧ください。